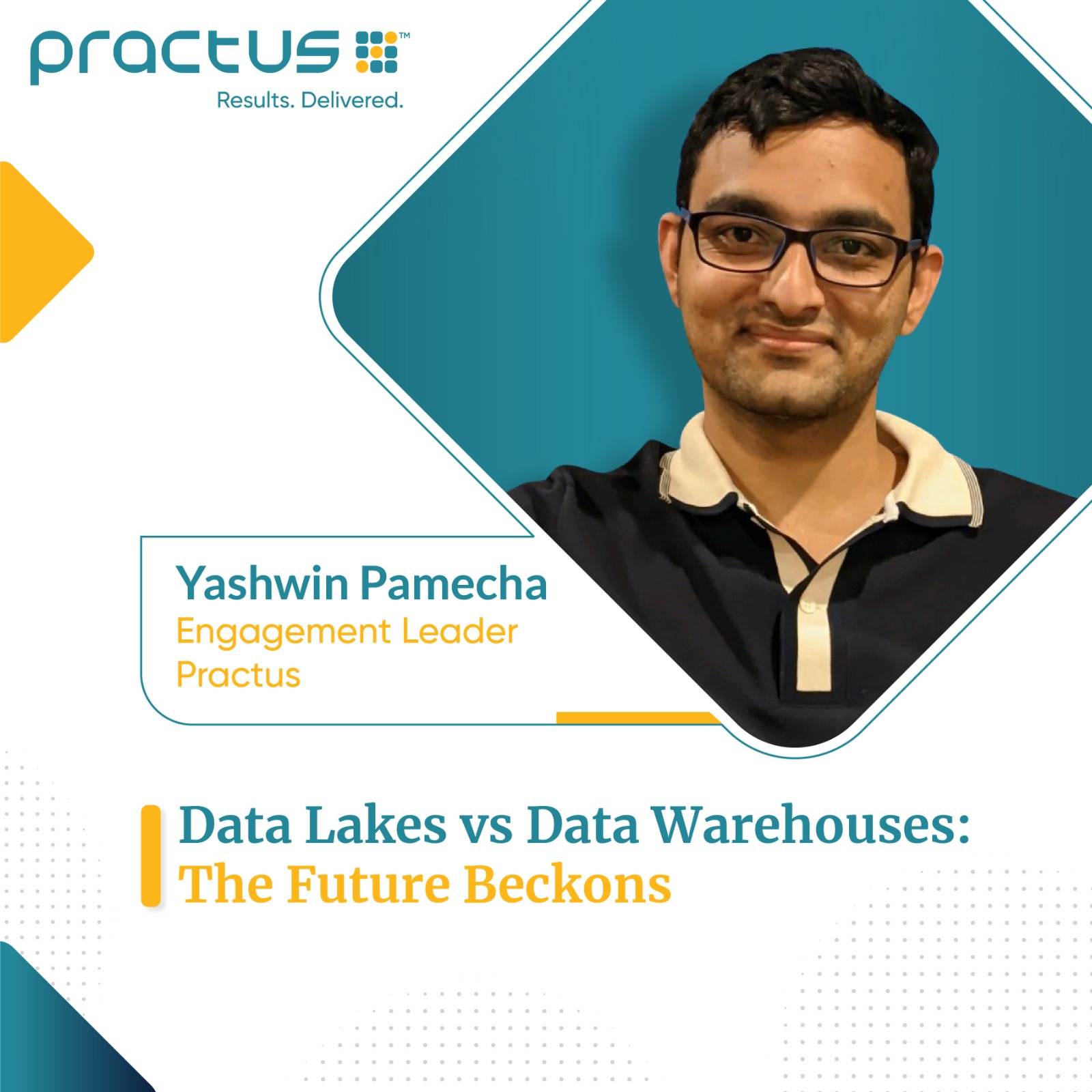
Data management is crucial to store and process the rapidly growing volumes of data efficiently. Data lakes and data warehouses are two pivotal data management solutions, both serving similar but distinct purposes. These competing technologies share a common goal of providing organizations with robust storage and processing capabilities.
The Metaverse and Its Potential Impact on B2B Interactions
The data lakes market is estimated to reach $37.76 billion. On the other hand, the data warehousing market is projected to reach $16.94 billion by 2029. The rapid growth of these markets is due to the rising demand for real-time data analytics and the increase in the use of Big Data applications. However, some major differences between data lakes and data warehouses make them suitable for different data types and use cases.
What is a Data Lake?
A data lake stores a vast amount of data in its native format. It acts as a large-scale holding center for data in various forms without requiring immediate purpose determination. Unlike traditional storage systems, a data lake does not require data to be structured before it is saved. A data lake segregates raw data as structured, semi-structured, or unstructured for storage. Data from various sources, including database records to Excel files, XML, photos, video content, and social media posts, is stored in raw, cleansed, and curated zones in the lake. The raw data can then be analyzed and processed using analytics tools to derive valuable insights. Data lakes offer scalable, cost-effective, and flexible data storage for easy processing. Data Lakes are capable of running real-time analytics, predictive modeling, machine learning (ML), and other intelligent data-driven decisions.
What is a Data Warehouse?
A data warehouse is a repository for structured historical data. It houses processed and refined data which can be quickly accessed to perform complex queries and analyses. They are designed to facilitate business intelligence activities, allowing data to be extracted, transformed, and loaded (ETL) for specific and strategic use. The process involves cleaning, standardizing, and transforming data for analysis. The unified data is then loaded into the warehouse for reporting. It is optimized for read operations with a specific schema designed to provide quick access to data using well-defined queries rather than ad-hoc analysis to extract insights.
Data Lakes vs Data Warehouses
Data Lakes and Data Warehouses are thought of as competing technologies, but they have distinct features. These data management solutions are suitable for different purposes. Here is a look at the key differences between Data Lakes vs. Data Warehouses:
| Data Lakes | Data Warehouses |
Data types | Stores all forms of data | Limited to structured and curated data |
Data processing | Designed for large volumes of raw data | Built for processing smaller curated sets |
Storage structure | Stores data in its original format | Follows a defined schema with tables |
Data purpose | Suitable for exploratory data | Holds data for business analysis/reporting |
Data access | Offers more flexibility in access/querying | Structured querying approach |
Cost | More cost-effective, no cleansing needed | Requires data cleansing and structuring |
Scalability | Infinite scalability for large data volumes | Limited capacity and complex to update |
The Future Needs More Manageable Data
Analytics is the cornerstone of informed decision-making. Organizations leverage technology to interpret enormous volumes of data, uncover hidden patterns, and gain critical insights for competitive advantage. The need for agile data management is driven by the demand for real-time analytics and the use of big data applications.
Data Analytics in 2024: 5 Key Trends Every Business Should Know
Data lakes offer more flexibility to manage the ever-expanding datasets required for comprehensive analytics, unlike data warehouses, which are optimized for speed during specific inquiries. Their ability to handle vast, varied, and unstructured data attributes provides the agility needed for advanced analytics. Moreover, data lakes offer lower-cost storage and are easier to scale.
The Rising Significance of Data Lakes in Analytics
One of the critical aspects of data lakes is their innate flexibility, as there is no need for immediate structure or schema. They can store any data regardless of its source, style, or structure until it needs to be used, refined, or discarded. It is highly advantageous for businesses to collect data from various sources and types. These diverse datasets, with their schema-on-read approach, are also helpful in training AI and ML algorithms for various use cases.
Data lakes facilitate real-time analytics as they do not require lengthy ETL processes. Due to the immediate availability of data in its raw form, analysts can perform exploratory and predictive analytics with minimal delay. It helps businesses stay competitive while ensuring the accuracy of business decisions.
Furthermore, data lakes accommodate growing volumes of data, enabling business agility and responsiveness. Their storage versatility makes them more cost-effective in the long run, as no upfront data cleansing and transformation tasks are involved. It is particularly beneficial for companies using Big Data techniques. They can store historical data for predictive analytics and data-driven decision-making.
Transitioning to Data Lakehouses
Data management cannot be a one-size-fits-all approach, as the amount of data stored by organizations is projected to reach 5.5 ZB by 2025. Data lakes can coexist with data warehouses, providing businesses with additional capabilities to make more informed decisions. The integration could help organizations achieve faster and more accurate insight.
A data lakehouse is an open data management architecture. It combines a data lake’s flexibility and scalability with a data warehouse’s structured querying approach. The hybrid approach supports ad-hoc analysis and pre-defined queries, providing more efficient and accurate data access. Data lakehouses are built on inexpensive and flexible storage technologies, such as cloud object storage, and support programming languages like Python, R, and high-performance SQL.
Data lakehouses support diverse workloads with features like ACID (Atomicity, Consistency, Isolation, and Durability) transactions, file caching, and indexing. It allows easy implementation of governance and security controls, reduces data duplication, and supports diverse workloads.
Data lakehouses are becoming a popular choice for businesses as they provide:
- Real-time analytics: Organizations can analyze data as it arrives and make timely decisions.
- Cost-effective: Robust features offered at low costs reduce the total cost of ownership.
- Scalable: Deals with vast volumes of data and scales based on business needs.
- Streamlined operations: Simplifies decision-making by providing a single source of truth for all critical data.
- Agile: Works with structured, semi-structured, and unstructured datasets to meet evolving business needs.
- Easy integration: Integrates with existing data lakes and data warehouses to provide a unified view of all data sources.
Data lakehouses simplify operational and management complexity. They are effective for users who need more data controls but do not need the full overhead of a separate data warehouse.
Key enablers of data lakehouses are:
- Metadata layers monitor open file formats (e.g., Parquet files) and track which files are part of different table versions for data validation. It offers rich management features like ACID-compliant transactions and enables schema enforcement and evolution.
- New query engine designs provide high-performance SQL execution. Data Lakehouses utilize techniques such as dynamic code generation and adaptive query optimization to provide faster performance over large and complex data.
- Access to data science and machine learning tools makes it easy to access the data in the lakehouse. The popular tools are pandas, TensorFlow, and PyTorch, which can already access sources like Parquet and ORC2.
Democratize your Data
Data availability is crucial for business efficiency and developing a collaborative organizational culture. Empowering individuals to access and utilize data effectively requires an architecture that makes data accessible to everyone. The adoption of data lakehouses has made it easier for organizations to make all their data available in one place and to all employees. The process is known as data democratization and enables the workforce to make data-informed decisions.
Key principles of data democratization are:
- Empower Employees: Enabling more employees to engage with data leads to better insights and informed decision-making.
- Provide the Right Data: Employees need different types or formats of data according to their workflow. However, most of it overlaps. According to McKinsey, there is a 50% overlap in the code base across industries. It shows most employees can use high-quality data sources like a Data Lakehouse.
- Perceive as an Ongoing Process: Data democratization is not a one-time event. It requires continuous effort from management, adoption of the latest technology, and an organization-wide mindset shift.
Embrace the data lakehouse to streamline data management and prioritize in-depth analytics as part of your core strategy. With their unrivaled capability to store and manage disparate data at scale, data lakehouses represent the future of data-centric businesses.
The Role of AI in F&A Operations